Energy crisis and environmental pollution are two major problems that threaten human’s healthy life and sustainable development. Large-scale promotion and development of new energy electric vehicles is one of the effective ways to solve the above problems. In recent years, under the background of the strategic goals of “carbon peak” and “carbon neutrality”, new energy vehicle technology has developed rapidly. Among all kinds of energy storage batteries, lithium-ion batteries stand out due to their high energy density, low self-discharge rate, and no memory effect, and have become the preferred energy storage components for electric vehicles.


In order to meet the voltage and power requirements of the load, the power battery pack is usually used in groups of hundreds or thousands of single cells. Due to differences in production technology, grouping methods, and heat dissipation conditions, there are inconsistencies in the parameters of voltage, current, and temperature when the cells are working, which can easily cause problems such as overcharging and over-discharging of some monomers, accelerate life decay, and affect the battery pack. Available capacity, cycle life, and even threaten system security. Therefore, the research on the life-cycle life decay mechanism of power lithium-ion battery system and the theoretical method of State of Health (SOH) estimation is an important cornerstone for the promotion and development of new energy electric vehicles.Lithium-ion battery SOH can be defined mainly from two perspectives: battery capacity attenuation and internal resistance increase, respectively mapping the attenuation of battery discharge capacity and the reduction of operating power. The current mainstream SOH estimation methods can be roughly divided into three categories: empirical/semi-empirical formula-based methods, model-based observer methods, and data-driven methods.The inherent disadvantage of methods based on empirical/semi-empirical formulas and model-based observer methods is their strong dependence on model accuracy, while data-driven methods combine historical and real-time data of actual battery operation with machine learning, neural networks, and other intelligent Algorithms are combined to estimate the SOH of the battery. Compared with the above two types of methods, the data-driven method tends to have stronger nonlinear approximation ability and has stronger applicability to different batteries and working conditions, but its prediction accuracy depends on the extracted aging features.Traditional feature extraction methods usually directly use data such as current and voltage during battery operation as aging features. This type of data has high measurement noise, and usually cannot directly and accurately characterize the aging state of the battery. Moreover, it requires the full profile of the battery features to start a relatively precise estimation of the SOH. In recent years, ICA (Incremental Capacity Analysis) curve analysis method for battery SOH estimation has received extensive attention. The IC (incremental capacity) curve is described as the incremental capacity over successive voltage steps and is obtained by comparing the capacity increment during the constant current charging phase with the voltage change. ICA method is capable of detecting and quantifying the degradation mechanism with the IC curves. Some researchers have applied Gaussian filter to effectively reduce the noise on IC curves for improving estimation results. Studies show that the IC curve of lithium-ion batteries contains many characteristics of battery aging and can further reflect the aging mechanism of batteries. ICA method has shown improved efficiency of estimation of SOH by extracting such characteristics without using the full profile and is very helpful for recalibrating the SOH.
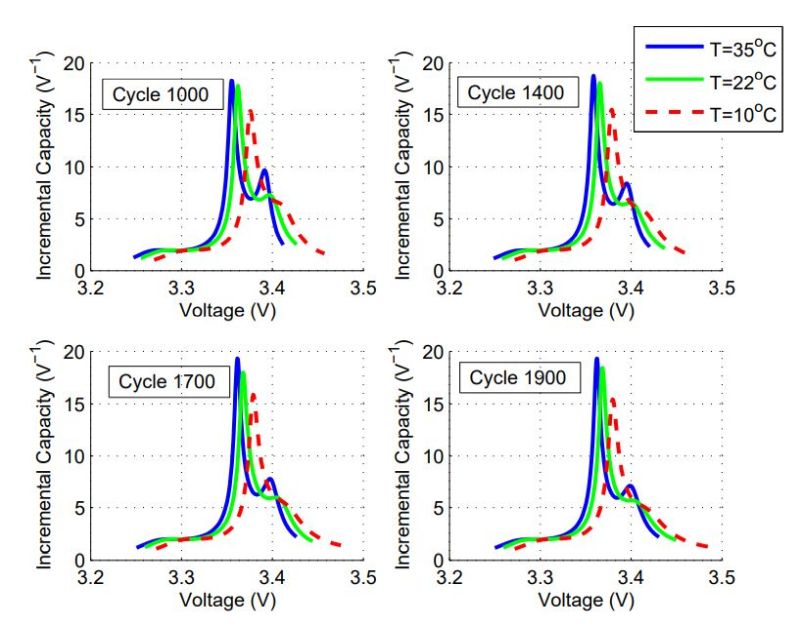

The health status of the battery is the core of battery health management. Accurate estimation of the health status of lithium-ion batteries is of great significance to ensure the safe, reliable and long-life operation of the battery.The ICA (Incremental Capacity Analysis) method extracts battery aging characteristics by analyzing the change mode of the charging increment capacity curve during the aging process of the battery. In order to reduce the computational complexity, the gray relational analysis method is introduced for feature analysis and screening, and it is used as the input of the long-short-term memory neural network for network pre-training to estimate the health status of the battery. The researchers finally verified the proposed health state estimation method using three different battery accelerated aging test datasets.Their experimental results show that the aging of lithium-ion batteries has a strong sensitivity to working conditions, and different charge-discharge rates have a greater impact on the aging rate of batteries. This method shows excellent battery health estimation performance, and in It shows good robustness under different working conditions and different training cycle numbers. By using ICA method for battery SOH estimation, the results have been improved greatly. Some studies show that the average estimation error is about 1%, and the maximum average error is no more than 4%. The following shows three different procedures for measuring SOH with ICA method.

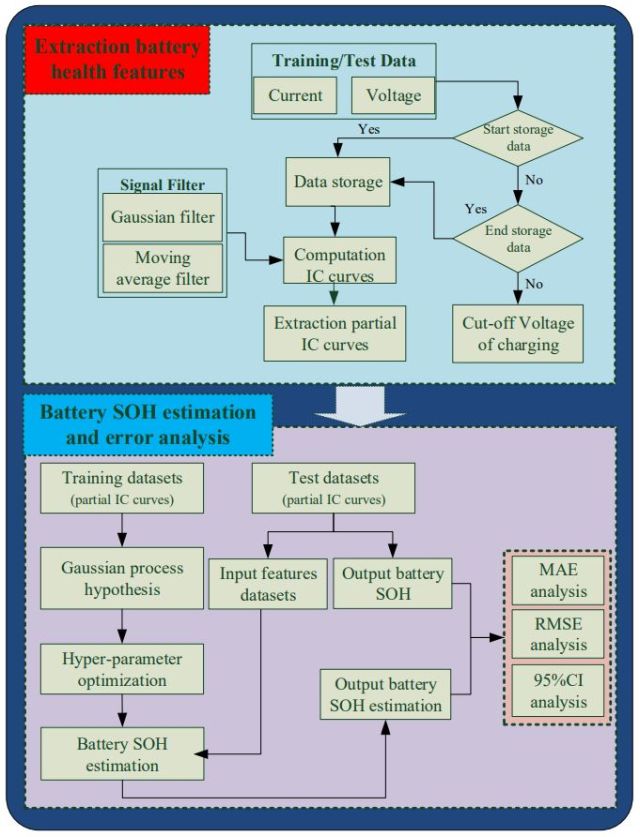

Reference
- Yao L, Wen J, Xu S, Zheng J, Hou J, Fang Z, Xiao Y. State of Health Estimation Based on the Long Short-Term Memory Network Using Incremental Capacity and Transfer Learning. Sensors. 2022; 22(20):7835. https://doi.org/10.3390/s22207835
- Han, X., Feng, X., Ouyang, M. et al. A Comparative Study of Charging Voltage Curve Analysis and State of Health Estimation of Lithium-ion Batteries in Electric Vehicle. Automot. Innov. 2, 263–275 (2019). https://doi.org/10.1007/s42154-019-00080-2
- Zhu, J., Wang, Y., Huang, Y. et al. Data-driven capacity estimation of commercial lithium-ion batteries from voltage relaxation. Nat Commun 13, 2261 (2022). https://doi.org/10.1038/s41467-022-29837-w
- P. Lin et al2020 J. Electrochem. Soc.167 090537DOI 10.1149/1945-7111/ab8f56
- Weng, C, Sun, J, & Peng, H. “An Open-Circuit-Voltage Model of Lithium-Ion Batteries for Effective Incremental Capacity Analysis.” Proceedings of the ASME 2013 Dynamic Systems and Control Conference. Volume 1: Aerial Vehicles; Aerospace Control; Alternative Energy; Automotive Control Systems; Battery Systems; Beams and Flexible Structures; Biologically-Inspired Control and its Applications; Bio-Medical and Bio-Mechanical Systems; Biomedical Robots and Rehab; Bipeds and Locomotion; Control Design Methods for Adv. Powertrain Systems and Components; Control of Adv. Combustion Engines, Building Energy Systems, Mechanical Systems; Control, Monitoring, and Energy Harvesting of Vibratory Systems. Palo Alto, California, USA. October 21–23, 2013. V001T05A002. ASME. https://doi.org/10.1115/DSCC2013-3979
- Jong, C. S. (2022). Incremental capacity analysis on module level li-ion batteries for echelon utilization (Bachelor’s thesis, University of Twente).
- Li, Y., Sheng, H., & Cheng, Y. (2020, October). Battery state of health estimation with incremental capacity analysis technique. In 2020 International Conference on Sensing, Measurement & Data Analytics in the era of Artificial Intelligence (ICSMD) (pp. 384-389). IEEE.
- Li, X., & Wang, Z. (2019). State of health estimation for lithium-ion battery by combining incremental capacity analysis with gaussian process regression. arXiv preprint arXiv:1903.07672.
- Lucas Albuquerque, Fabien Lacressonnière, Xavier Roboam, Christophe Forgez. Incremental Capacity Analysis as a diagnostic method applied to second life Li-ion batteries. ELECTRIMACS 2022, 14th International Conference of the International Association for Mathematics and Computer in Simulation, May 2022, Nancy, France. ⟨hal-03673979⟩
- Si-zhe Chen, Hongtao Zhang, Long Zeng, Yuanliang Fan, Le Chang, and Yun Zhang, Secondary Structural Ensemble Learning Cluster for Estimating the State of Health of Lithium-Ion Batteries, ACS Omega 2022 7 (20), 17406-17415, DOI: 10.1021/acsomega.2c01589
- Krupp A, Ferg E, Schuldt F, Derendorf K, Agert C. Incremental Capacity Analysis as a State of Health Estimation Method for Lithium-Ion Battery Modules with Series-Connected Cells. Batteries. 2021; 7(1):2. https://doi.org/10.3390/batteries7010002